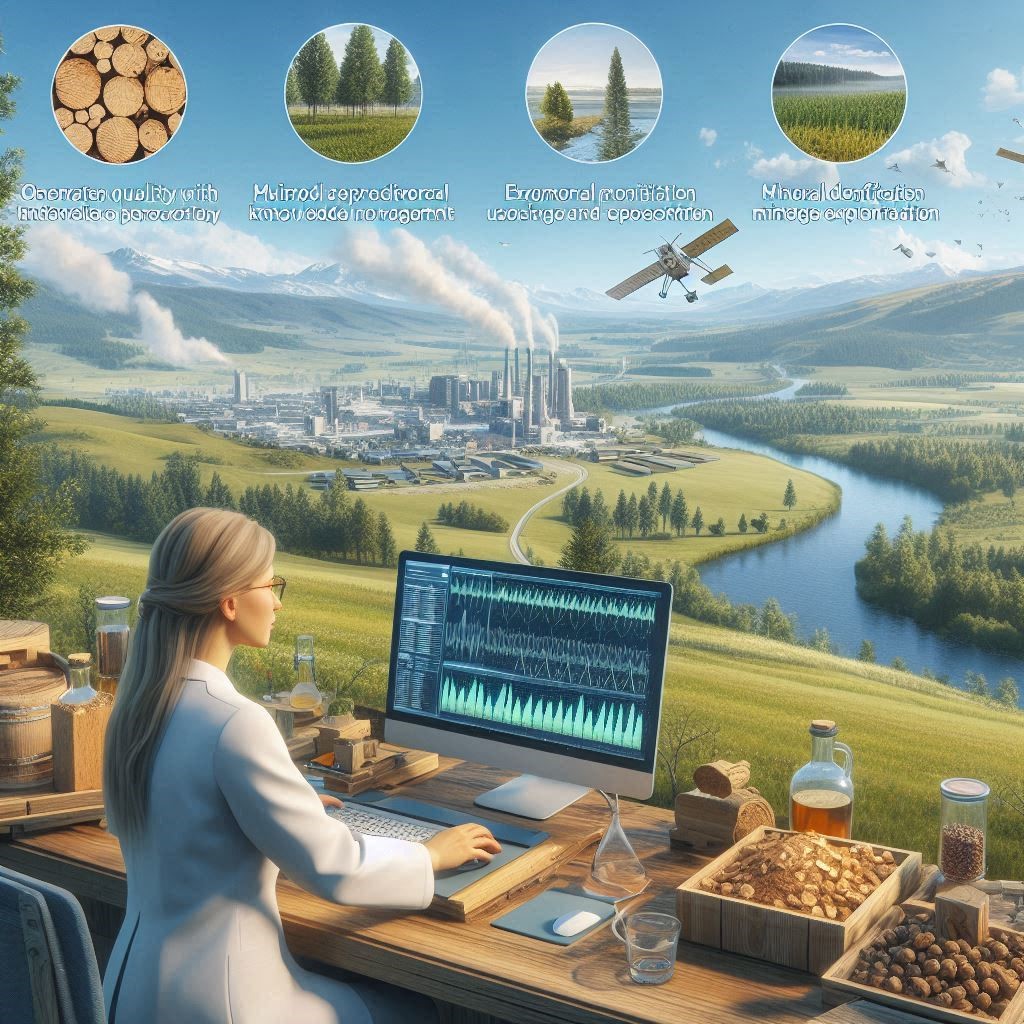
The present invention discloses a method of multimodal spectral imaging that utilizes a knowledge distillation framework to implement a new technique for the classification of unknown samples called Multimodal Spectral Knowledge Distillation (MSKD). In general, it consists of a processing pipeline and its algorithm implementation that presents an innovative approach to sensor fusion in the context of spectral imaging.
It uses one of the sensing modalities as an autonomous supervisor of the others, allowing to achieve higher performances increasing the sizes of the training datasets.
Spectral imaging is widely used in industrial processes like material sorting and metallurgy process control using LIBS, and food safety, quality control, and mineral exploration using HSI. However, these methods have limitations such as inconsistent results and a lack of interpretability. Particularly, developing reliable solutions and overcoming the complex nature of hyperspectral data calls for innovative approaches to feature extraction and data interpretation.
Multimodal spectral imaging solutions combine information from different sources to enhance single modality systems. But finding effective solutions is challenging due to the need for labeled training data, which is currently highly dependent on manual labelling, and hinders its overall performance. This gap creates an opportunity for developing an automatic and effective method for real-time mineral identification.
SpectralKD is a new approach to collaborative sensing that uses a multimodal knowledge distillation technique to enhance the performance of a data-driven model. It does this by using a two-step autonomous supervision framework that trains an unsupervised classification model using one spectroscopy technique to provide reliable labels (teacher). Then, the second technique (student) is trained in a supervised manner using the reliable labels from the first step, resulting in improved performance. SpectralKD capitalizes on the multimodality through the teacher technique and overcomes the challenges of finding labeled training data.
The technology provides an automatic and effective way for real-time mineral identification, which can bring significant benefits to multiple industry sectors. Among its main benefits are: Automation - allows the design of autonomous data-driven solutions for mineral identification, enabling fast decision making in the operational scenarios; Cost-Efficiency - Fast decision-making in operational scenarios independent of intensive and tedious manual labeling; Customizable - able to construct/teach training datasets for specific problems in a time efficient manner; Flexible - can be used with different sets of teacher/student spectral techniques; Improves accuracy and interpretability of the "student" technique - using teacher techniques to generate trustworthy labels for the student and increase performance and transparency; Sustainability - this approach provides a way of maintaining the performance of the knowledge distillation solutions over the time without expert knowledge.
In process control in cork industry and wood recycling; Environmental monitoring and resource management using remote sensing; Mineral identification in mining operations such as prospection and exploration;